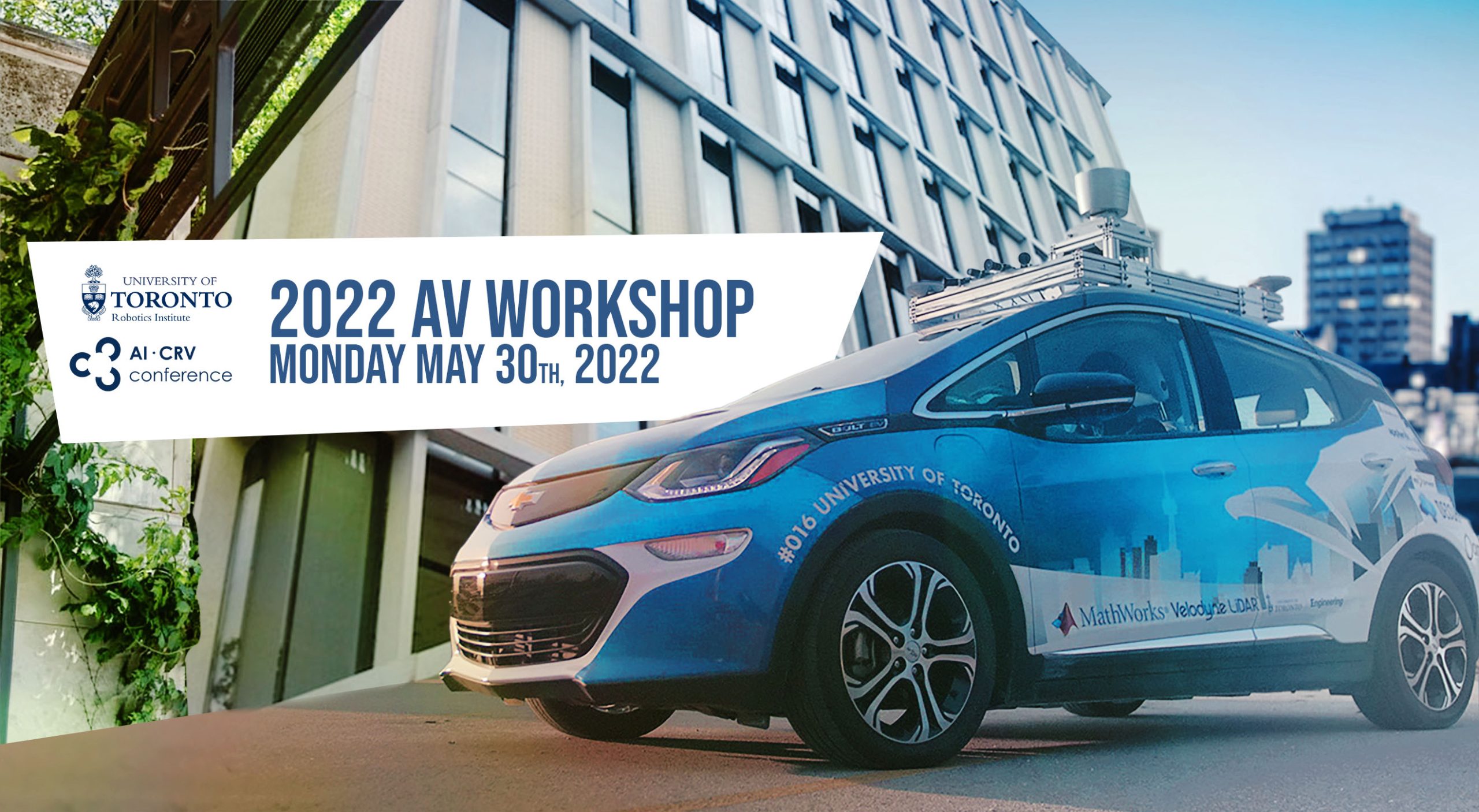
Sponsored by
Autonomous Vehicles Workshop
Co-located with this year's Artificial Intelligence conference and Conference on Robots and Vision (AI CRV 2022), this workshop focuses on the latest innovations in Autonomous Vehicles being developed at the University of Toronto Robotics Institute. Faculty and student researchers from a range of faculties will present their latest work on autonomy, safety and reliability of self-driving systems.
Monday, May 30, 2022
Times listed in Eastern time zone
Time | Activity | |
---|---|---|
11:00 | Welcome: Steve Waslander | |
11:10 | Keynote Speaker: Ben Upcroft, VP Technology, Oxbotica | |
12:15 | Coffee | |
12:30 | Student Session: 6 x 7 minute talks + 3 minutes for questions | |
13:30 | Lunch | |
14:30 | Faculty Speaker: Tim Barfoot - Working a Crowd: Learning to Navigate Crowded Indoor Spaces | |
14:45 | Faculty Speaker: Ben Wolfe - The Human Side of the Equation: What Does the Driver Need to Know | |
15:00 | Faculty Speaker: Sanja Fidler | |
15:15 | Blitz Panel: Getting to Level 5, if or when? | |
15:30 | Coffee | |
15:45 | Faculty Speaker: Igor Gilitschenski - Playing by the Rules: Learning Behavior Models for Autonomous Driving | |
16:00 | Faculty Speaker: Steve Waslander - All-Weather Autonomous Driving: The WinTOR Program | |
16:15 | WinTOR Students: 3 x 7 minute talks + 3 minutes for questions | |
16:45 | Closing Remarks: Jonathan Kelly |
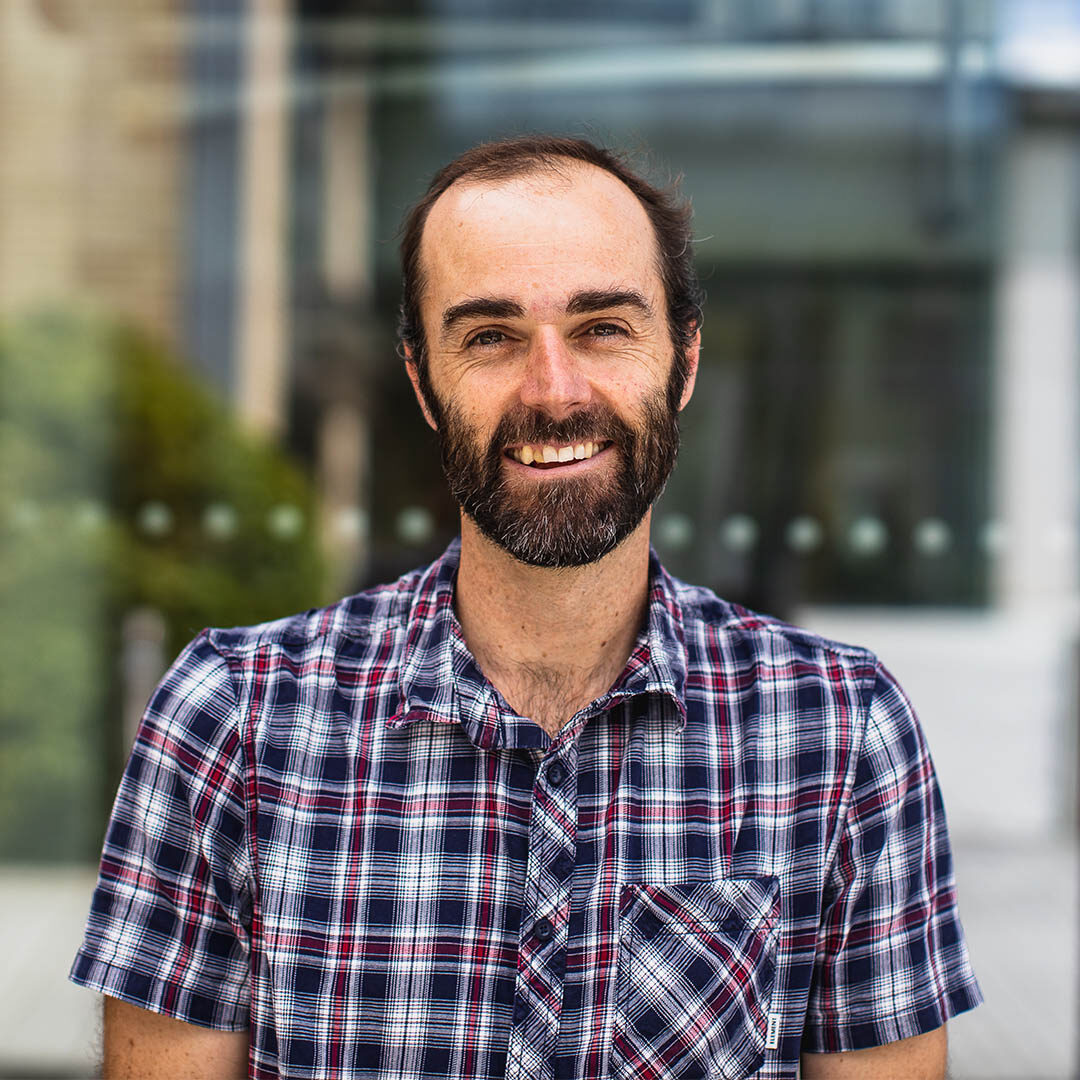
Keynote Speaker: Ben Upcroft - Oxbotica
VP of Technology, Oxbotica
Ben is the VP of Technology at Oxbotica, with extensive experience in perception systems for field robotics ranging from commercial passenger vehicles, all-terrain vehicles, draglines, haul trucks, underwater platforms, to unmanned aerial vehicles.
At Oxbotica, Ben is responsible for technology development and our team of skilled engineers, balancing state of the art algorithms with real world system implementation to achieve intelligence in self-driving platforms - on and off road.
Ben's previous life was as a tenured academic in Computer Vision and Robotics at the Queensland University of Technology, Australia where he focussed on machine learning for field robotics. He led the Robotics and Autonomous Systems group consisting of over 150 postgraduate students, postdocs, and academics.
Abstract
Ben will give an overview of Oxbotica and what Oxbotica does (this might not be so obvious as you think). He will discuss the different domains (including ports, mines, quarries, airports, refineries, solar farms, urban road, cities) that Oxbotica's software is deployed into and how we've developed software that is agnostic to the domain, to the vehicles Oxbotica runs on, and to the sensors that are used. Ben will also give some insight into the challenges that they have had to overcome and the ones yet to be faced.
Faculty Speaker: Sanja Fidler
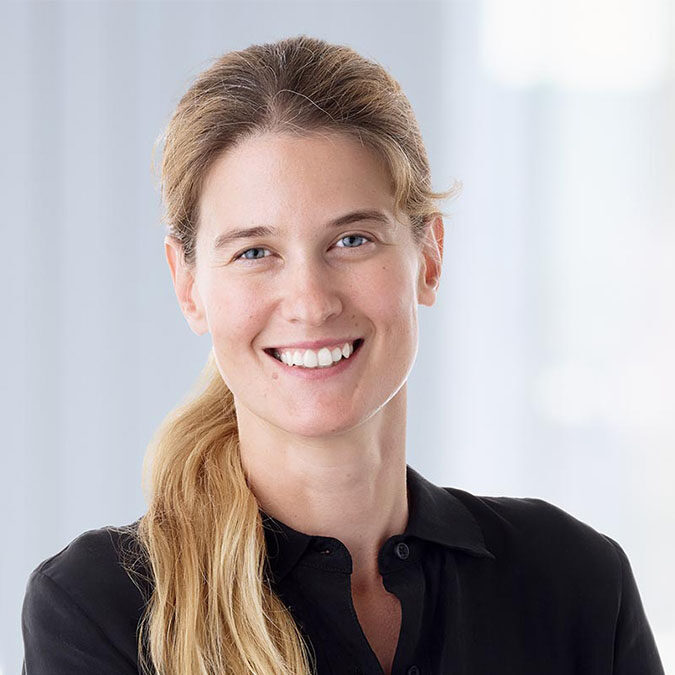
Prof. Sanja Fidler is an Associate Professor in the Computer Science Department at the University of Toronto and a Director of AI at NVIDIA. Prior to coming to Toronto, in 2012/2013, she was a Research Assistant Professor at the Toyota Technological Institute at the University of Chicago. Her research interests lie in the area of Computer Vision. Her main research interests are 2D and 3D object detection, particularly scalable multi-class detection, object segmentation and image labeling, and (3D) scene understanding. She is also interested in the interplay between language and vision: generating sentential descriptions about complex scenes, as well as using textual descriptions for better scene parsing (e.g., in the scenario of the human-robot interaction).
Faculty Speaker: Tim Barfoot
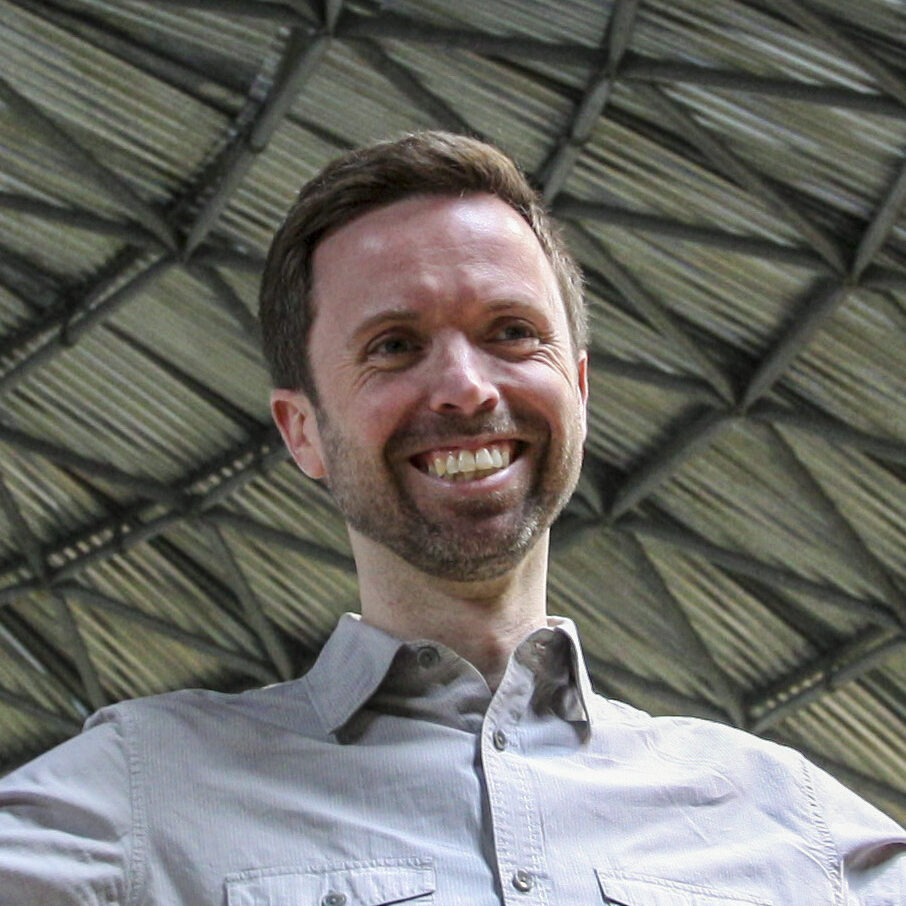
Prof. Timothy Barfoot (University of Toronto Institute for Aerospace Studies – UTIAS) works in the area of autonomy for mobile robots targeting a variety of applications. He is interested in developing methods (localization, mapping, planning, control) to allow robots to operate over long periods of time in large-scale, unstructured, three-dimensional environments, using rich onboard sensing (e.g., cameras and laser rangefinders) and computation. Prior to UTIAS, he was at MDA Robotics (builder of the well-known Canadarm space manipulators), where he developed autonomous vehicle navigation technologies for both planetary rovers and terrestrial applications such as underground mining. Along with many accolades, he is the author of State Estimation for Robotics (2017), which is free to download from his webpage.
Title: Working a Crowd: Learning to Navigate Crowded Indoor Spaces
Abstract: Many environments such as offices, shopping malls, airports, and tourist attractions are quite dynamic yet lack structure constraining the motion of people, strollers, carts, and other moving elements. I will discuss an approach we have been developing to let mobile robots navigate in a more socially aware manner. We take a lifelong learning approach to the problem where we eschew the use of human-labelled training data and instead attempt to learn what “stuff” can move and how it can move using purely self-supervision. We leverage an offline multi-session SLAM algorithm to annotate previous navigation experiences, then train networks to make fast predictions online. We show how our learned predictions can be used to improve navigation both in simulation and hardware experiments. We believe this generic paradigm of self-supervised annotations and lifelong learning to be a scalable approach that can be applied to a number of mobile robot navigation domains.
Faculty Speaker: Ben Wolfe
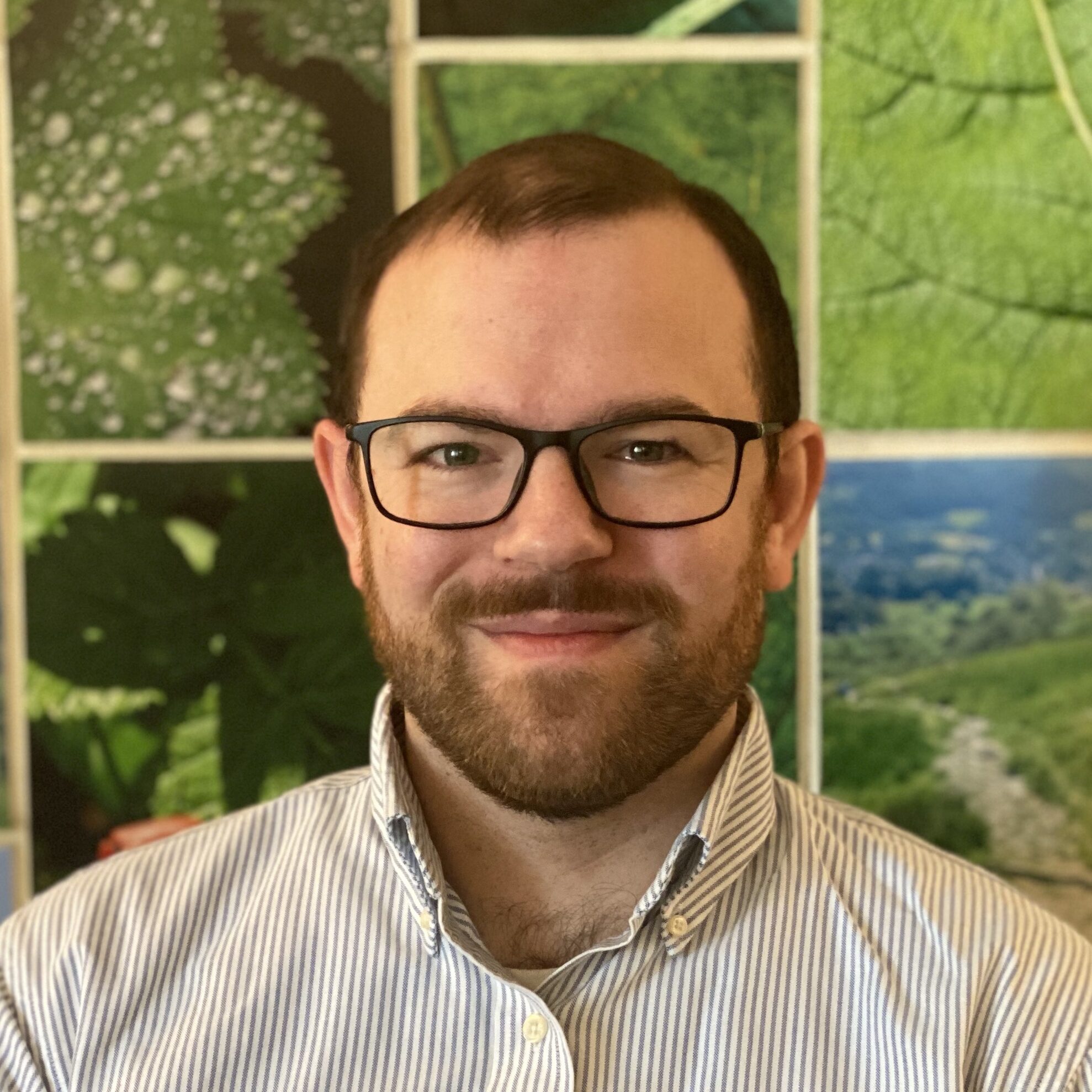
Dr. Benjamin Wolfe is a Director of the Applied Perception and Psychophysics Lab (www.applylab.org) at the University of Toronto, which examines questions in driver behaviour in terms of human visual perception, aiming to answer the question of what the driver needs to know and how they acquire visual information, necessary to build safe cars today, and autonomous vehicles which understand drivers' limitations in the future. His work has previously been funded by the Toyota Research Institute and NSERC, and takes a use-inspired approach to studying these timely problems.
Title: The human side of the equation: What does the driver need to know, and how do they know it?
Abstract: Autonomous vehicles promise to take the human out of the equation, but until they entirely replace human drivers, we need to understand how they acquire and represent information about the operating environment. Using tools and techniques from vision science and psychology, I will discuss results demonstrating how quickly drivers acquire information about the road environment and how this varies by driver age. I will also talk about some of the frightening errors that humans make on the road, how we might try to ameliorate them, and why knowing these strengths and limitations is essential to build safer cars now, and more capable autonomous vehicles in the future.
Faculty Speaker: Igor Gilitschenski

Faculty Speaker: Steve Waslander
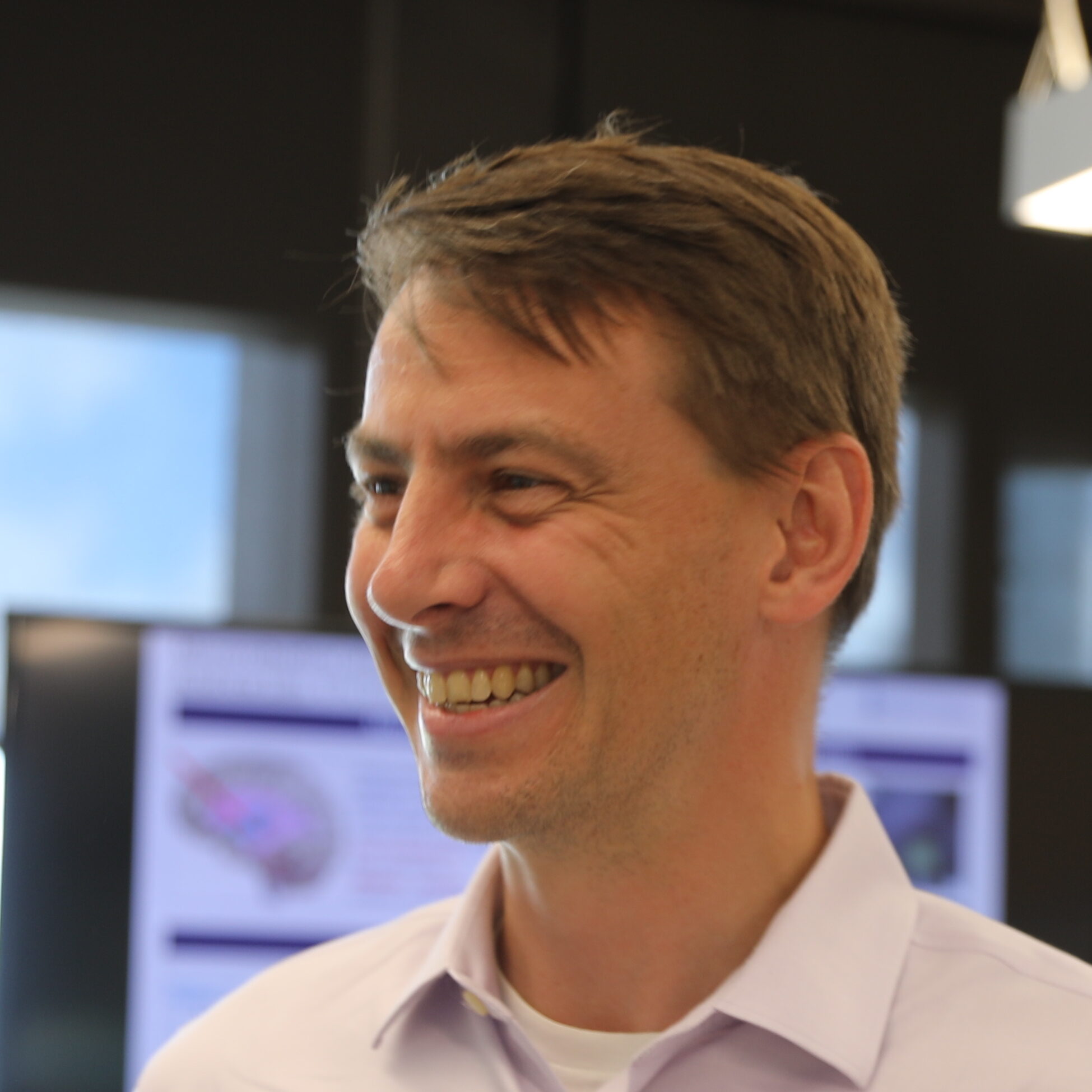
Prof. Steven Waslander is an Associate Professor at the University of Toronto Institute for Aerospace Studies and an Adjunct Professor in the Mechanical and Mechatronics Engineering department, and the former Director of the Waterloo Autonomous Vehicles Laboratory (WAVELab). He is a leading authority on autonomous aerial and ground vehicles, including multirotor drones and autonomous driving vehicles. His research interests include Multirotor Drones, Aerial Robotics, Robotic Vision, SLAM, Scan Registration, Motion Planning, Autonomous Driving. Professor Waslander is a member of the NSERC Canadian Field Robotics Network. He also acted as the academic advisor to the University of Waterloo Robotics Team, which both compete in a large number of competitions such as the NASA Sample Return Robot Challenge, the Intelligent Ground Vehicle Competition, and the International Autonomous Robot Racing competition.
Title: All-Weather Autonomous Driving: The WinTOR Program
Abstract: The wide variation of weather conditions encountered while driving result in many challenges to the reliable autonomy of the driving task. Sensor data can be seriously degraded, leading to significant reductions in perception and localization performance. Driving behaviours change dramatically, requiring adaptability and coordination in prediction and interaction planning. Control authority can be both significantly reduced and highly variable, requiring robust control techniques and safe learning strategies. The WinTOR project has been designed to address these issues and will advance the state of the art in perception, localization, planning and control for adverse weather conditions through a five-year collaborative research project supported by the Ontario Ministry of Colleges and Universities, together with partners GM Canada, LG Electronics, Applanix and Algolux.
Student Session
(6 x 7 minute talk + 3 minute questions)
Moderation: Igor Gilitschenski
Talk 1: Frank Qian (aUToronto), "aUToronto Year 5: A New Perception Challenge"
Talk 2: Mona Gridseth (ASRL), "Deep learned features for long-term visual localization"
Talk 3: Andrei Ivanovic (TISL), "Coordinated Multi-Agent Motion Planning via Imitation Learning"
Talk 4: Jonah Philion (UofT, NVIDIA), "Self-Driving Encoders and Decoders"
Talk 5: Sandro Papais (TRAIL), title "Sliding Window 3D Multi-Object Tracking for Autonomous Vehicles"
WinTOR Student Session
(3x 7 minute talk + 3 minute questions)
Moderator: Steve Waslander
Talk 1: Barza Nisar, " Perception in all types of Precipitation"
Talk 2: Keenan Burnett, "Are We Ready for Radar to Replace Lidar in All-Weather Mapping and Localization?"
Talk 3: Jordan Hu, "SampleNet: Particle-based Uncertainty Prediction with Application to Monocular Depth Estimation"
About WinTOR
Northern climates introduce a significant additional barrier to entry for autonomous vehicles and advanced driver-assistance systems due to the increased complexity of vehicle perception, motion prediction and vehicle control during winter driving. While the initial race to market is on, this added hurdle poses a significant additional challenge beyond the steep barrier of demonstrable autonomous safety. Hence, it is not currently a priority for most major players in the autonomous vehicles space. However, it is clear that fundamental research into robust and uncertainty aware algorithms is still needed to maintain the high safety requirements expected of autonomous vehicles in all driving conditions. We are accelerating work in this domain to ensure self-driving cars are ready to meet any challenge they encounter in a dynamic world.