Upcoming Seminars
2024-25 series coming soon.
Past Seminars
JULY 9, 2024

Robert Gregg
University of Michigan
Enhancing human mobility with agile robotic prostheses and orthoses
APRIL 5, 2024

Fabio Ramos
University of Sydney
Probabilistic Robotics 2.0: Leveraging Differentiability and Parallelism for Diversity in Planning and Perception under Uncertainty
MARCH 22, 2024

Hao Dong
Peking University
Towards Unified Robotics Manipulation via Object-centric Policy
MARCH 8, 2024

Zachary Manchester
Carnegie Mellon
Composable Optimization for Simulation, Motion Planning, and Control
JAN 26, 2024

Noémie Jaquier
Karlsruhe Institute of Technology
From Data Structure, Physics and Human Knowledge: A Manifold of Robotic Geometries
DECEMBER 8, 2023
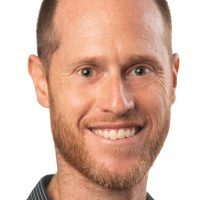
Oren Salzman
Technion — Israel Institute of Technolgy
The multi-objective shortest-path problem: State-of-the-art algorithms with applications to robot motion planning
OCTOBER 13, 2023

Stefana Parascho
EPFL
From Automation to Collaboration: Repositioning Robotics in Construction
OCTOBER 20, 2023
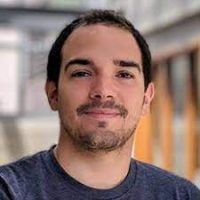
SEPTEMBER 29, 2023
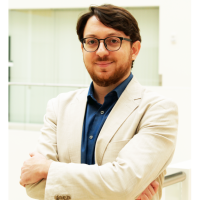
Luca Carlone
MIT
Next-Generation Robot Perception: Hierarchical Representations, Certifiable Algorithms, and Self-Supervised Learning
JULY 10, 2023

David Gracias
Johns Hopkins University
Smart 3D Microtechnologies for Biology and Human Health
June 13, 2023
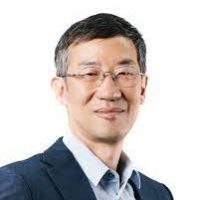
Frank Park
Seoul National University
Geometric Methods for Robot Learning: From Models to Representations
MAY 10, 2023
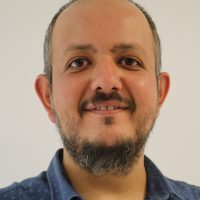
Onur Ozcan
Bilkent University
Miniature Bio-Inspired Robots: Rigid to Compliant
APRIL 21, 2023
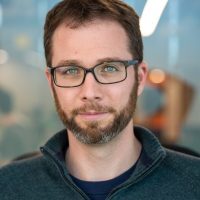
Dmitry Berenson
University of Michigan
Where to Trust and How to Adapt Learned Models for Motion Planning
MARCH 10, 2023
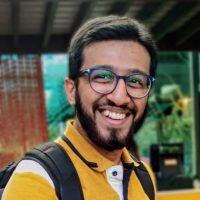
Mustafa Mukadam
Meta
Theseus: A Library for Differentiable Nonlinear Optimization
FEBRUARY 17, 2023
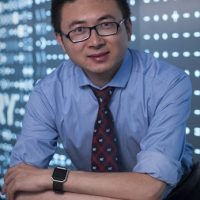
FEBRUARY 10, 2023

Arianna Menciassi
Scuola Superiore Sant'Anna
Robotics and Bioengineering for Minimally Invasive Surgery and Targeted Therapy
JANUARY 13, 2023
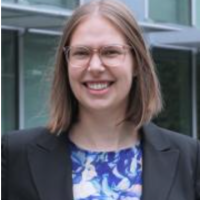